Assessing Prediction Accuracy of Machine Learning Models
- ケース
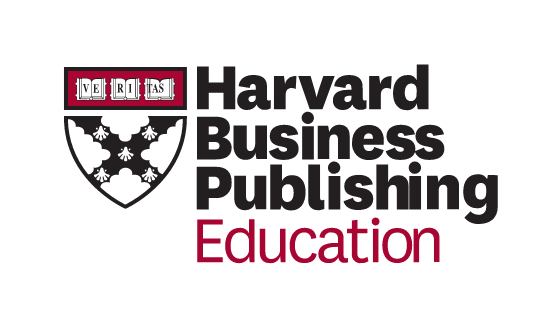
The note introduces a variety of methods to assess the accuracy of machine learning prediction models. The note begins by briefly introducing machine learning, overfitting, training versus test datasets, and cross validation. The following accuracy metrics and tools are then described: mean squared error (MSE), mean absolute deviation (MAD), Brier score, and cross-entropy, true/false positives/negatives, the confusion matrix, true positive rate (sensitivity or recall), false negative rate (Type II error rate), precision, true negative rate (specificity), false positive rate (Type I error rate), receiver operating characteristic curve (ROC) and area under the curve (AUC), and precision-recall curve.
- 出版日
- 2020/08
- 改訂日
- 2020-09-01
- ボリューム
- 12ページ
- コンテンツID
- CCJB-HBS-621045
- オリジナルID
- 621045
- ケースの種類
- Case
- 言語
- 英語
- カラー
- 製本の場合、モノクロ印刷での納品となります。